How can we use artificial intelligence (AI) to improve drug discovery and development? In this installment of our deep dive, we describe how AI and machine learning (ML) can improve chemical synthesis R&D, an area of drug development where pharmaceutical companies have struggled with in recent years. We also introduce several startups using AI to plan and automate chemical synthesis.
The Current State of Early Drug Discovery
1 in 20,000: The Drug Development Lottery
Drug development is the process of transforming a molecule from a drug candidate—the end-product of the discovery phase—into a marketable product that has been given the green light for commercialization by the relevant regulatory authorities. These include the FDA in the U.S., EMA in the E.U., NMPA in China and PMDA in Japan, to name a few.
On average, for every 10,000 compounds screened in the discovery phase, only 250 will pass through to more rigorous preclinical studies. Eventually, only 5 of these 250 will be deemed worthy of highly regulated clinical trials (usually consisting of 3 phases). Now let’s take a look at the success rate of drugs that pass clinical trials and enter the market.
Cancer (oncology) drugs comprise 31% of all clinical trials. Still, they have an overall success rate of just 5.1%, a very low figure considering the enormous costs associated with the latter stages of drug development. When we exclude this class of drugs, the overall success rate of drugs that enter clinical trials goes up to 11.9%.
This means that only 1 in ~20,000 compounds screened will become an actual drug product! Including the costs of covering 19,999 failures, the drug development process takes 10 to 15 years and costs on average US$2 billion for every new drug that enters the market.
The Decline of Pharmaceutical R&D
Drug development is a lengthy, complex, inefficient and capital-intensive process that involves significant outlays by the pharmaceutical industry and national governments (who provide grants and loan guarantees). To compound this, a drug candidate will likely fail somewhere along the line and the entire process has to restart from square one.
And the process doesn’t seem to be improving. Pharma R&D productivity has been declining since 1950, with the number of new medicines for every US$1 billion spent halving every 9 years since. A 2017 study of 30 pharmaceutical and biotech companies found that drugs developed within the past five years only accounted for a measly 11% of revenue, with older, more established drugs making up the bulk of their inlays.
In another 2018 report, the projected R&D returns on investment of a cohort of 12 biopharma companies reached a new low of 1.9%, nearly half of the 3.7% ROI in 2017 and a drastic year-on-year decline since a high of 10.1% in 2010.
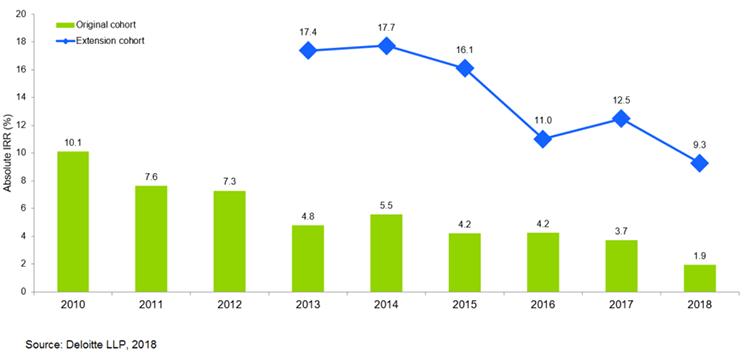
According to the same report, this decline in projected returns can broadly be broken down into rising R&D costs to develop an asset from discovery to launch, and declining forecast peak sales from pipeline assets once launched.
Plotting the Pharma Industry’s Great Escape
While there are many non-technical factors involved in the R&D process, it is possible that one (or a blend) of the technological innovations and megatrends highlighted below could play a key role in helping the pharmaceutical industry out of this rut.
- Artificial Intelligence (AI)/Machine Learning (ML): A set of AI-powered solutions and ML technology with potential applications in every drug development process.
- Blockchain: A worldwide decentralized and public digital ledger that records transactions on many computers so that no record can be altered retroactively) is an excellent technology for protecting confidential data even in healthcare. For example, this technology can help healthcare researchers to understand genetic codes, to manage the drug supply chain or to facilitate the safe transfer of a patient’s medical records.
- Quantum Theory: If you think AI/ML is complicated wait until you see the fundamental laws of quantum mechanics like “If you tickle one…both will laugh: the law of quantum entanglement”. It may sound strange, but quantum principles like retrocausality and determinism can be applied in healthcare for imaging microscopes, entangled proteins, and of course in quantum computing to simulate more extensive and more complex models for drug discovery.
- Microbiome: Using bioinformatics to understand how the body’s trillions of microorganisms work together in processes like metabolism and the immune response is emerging as a critical player in drug development. Using microbiome technology, we can develop better drugs for various diseases including diabetes, liver diseases, tumors and pathogen infections.
In particular, AI/ML is predicted to change health care by advancing drug development and clinical research. Therefore, to make the drug development process more efficient hundreds of new data-driven startups are working right now from the discovery and preclinical phase to the clinical phase, trying to optimize every single step of drug development using AI/ML tools.
Applying Artificial Intelligence and Machine Learning to Drug Development
AI/ML Tools by the Numbers
Before we go into the technical side of artificial intelligence, let’s look at the tangible benefits of using this technology in drug development. According to an Insider study, by using the different AI/ML tools that are now available, a company can make more accurate predictions about how drugs might treat diseases. With this targeted knowledge, they predict these tools can lower drug discovery costs by nearly 70%!
On top of that, research biotechnology analysts from Morgan Stanley estimate pharmaceutical companies can experience a 20% to 40% reduction in costs by implementing AI/ML technologies in just the preclinical development stage.
Applying these cost savings across a subset of US biotech companies, it is estimated that the amount saved could fund the successful development of four to eight novel molecules into drugs annually. That means more drugs to treat diseases, ultimately benefiting more patients.
Which Parts of Drug Development Can Be AI-Assisted?
Given the importance of artificial intelligence in early drug development, we highlighted in our previous article several AI/ML tools and startups that are currently involved in accelerating primary and secondary screening. These helped in the process of screening molecules for suitability as a new drug candidate (or lead compound), involving exploring vast chemical libraries using high throughput in vitro and in silico studies and secondary assays.
However, drug screening is just the tip of the iceberg of AI possibilities; other applications include:
- medicinal chemistry for design and synthesis of the new drug candidate
- optimization of hits to reduce potential drug side effects, and
- in silico studies combined with cellular functional tests are used to improve the functional properties of the drug candidates.
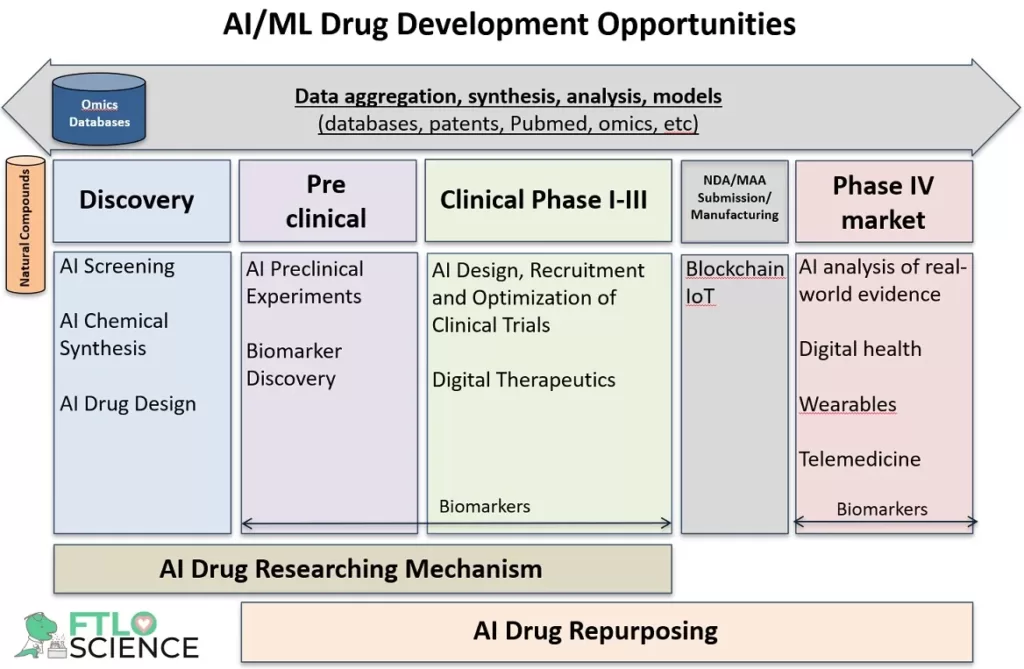
As we continue our journey through this fantastic and emergent AI/ML drug discovery landscape, this article will focus on some of the AI/ML tools and startups for planning and execution of chemical synthesis during drug discovery.
AI in Chemical Synthesis: Research and Planning
What is Chemical Synthesis in Drug Discovery?
Chemical synthesis lies at the heart of developing molecules into medicines. After all, molecules don’t grow on trees (actually, many drug precursors do, but that’s not the point), so we need a reliable way to perform our own chemical reactions in laboratories and manufacturing plants to ensure a supply of drug products.
From a drug development perspective, chemical synthesis planning begins after identifying a suitable lead compound using drug screening techniques. However, many of these compounds exist only in chemical libraries and do not have a reliable or optimal chemical synthesis pathway.
Developing such synthetic pathways is traditionally the realm of organic chemists, who study a target molecule and dream up reaction mechanisms to synthesize it quickly and efficiently.
Working Backwards with Retrosynthesis
Since we often already have the final chemical structure in mind, we can perform what’s known as retrosynthetic analysis (or retrosynthesis), namely tracing the steps in reverse. Retrosynthesis is considered a cornerstone of organic chemistry, which can involve planning a complete synthesis pathway to create complex organic molecules found in nature using only simple precursors (sometimes called total organic synthesis).
In retrosynthesis analysis, the target compound is first reduced into a sequence of progressively simpler structures, aiming to identify a simple or commercially available starting material to work with. By exploring different pathways, retrosynthetic analysis can generate a variety of possible starting materials from which we can synthesize the lead compound.
While this sounds great in theory, the reality is that retrosynthesis analysis is highly challenging. 60% of all FDA-approved drugs are naturally occurring compounds or their derivatives, yet most biosynthetic pathways remain unknown. This means that we must obtain the ingredients for these drugs from natural sources, making their manufacture expensive and sometimes unsustainable.
This is where AI and computer-aided tools come in handy. If we want to create an artificially “intelligent” tool for the planning and execution of chemical synthesis, the tool must be able to:
- Think like a human and perform tasks on its own, and
- Possess neural networks (a series of algorithms that attempt to mimic the human brain) so that such intelligence can be improved through logical reasoning, via machine learning (ML).
The Monte Carlo Tree Search (MCTS) in Retrosynthesis Research
Since ML requires an existing knowledge database to improve its decision-making skills (hence “learning”), we must first obtain existing retrosynthesis information of related molecules (cataloged in libraries such as the Dictionary of Natural Products).
Once the system has been “trained”, we can present previously unstudied drugs to the same system to develop chemical synthesis pathways for them, using the Monte Carlo tree search (MCTS) technique.
MCTS is an algorithm in the field of AI that figures out the best move out of a set of moves to generate a final solution. Given a set of inputs (in this case, chemical building blocks), MCTS can assess the possible pathways of putting them together to generate the target compound.
These building blocks are known as nodes, which gradually expand the ‘tree’ as more nodes are added to the algorithm. As we perform more searches using the same nodes, the tree grows in size as well as in knowledge, which means that we can repeat the search to receive better and more probable outcomes.
MCTS is probabilistic and heuristic; it is based on statistics alone and does not require any strategic or tactical knowledge about the given domain to make reasonable decisions. This form of search algorithm is helpful for making sequential truncation predictions such as in retrosynthesis, combining the classic tree search alongside ML principles of reinforcement learning.
Combining MCTS with Reinforcement Learning
Reinforcement learning (RL), is an area of ML concerned with how intelligent agents should act in an environment to maximize the cumulative reward. Essentially, RL is based on interactions between an AI system and its environment and helps assess whether an algorithm produces the correct answer. RL is one of the three basic ML paradigms, alongside supervised learning and unsupervised learning.
Furthermore, RL can be implemented using neural networks, allowing the system to assess outcomes using existing knowledge. For example, by supplying known retrosynthesis information, we can train neural networks to evaluate the viability of a proposed chemical synthesis pathway.
By combining MCTS with three neural networks, a method known as 3N-MCTS is reported to generate retrosynthesis pathways and evaluate their feasibility. This computing system was inspired by biological neural networks that consist of three layers: an input layer, a hidden layer and an output layer.
The 3N-MCTS method offers a workflow for computer-assisted retrosynthesis by evaluating the feasibility of a chemical transformation, having been trained using 12.4 million transformation rules from existing organic synthesis literature. Additionally, the speed of retrosynthesis prediction per molecule with 3N-MCTS is, on average, 20-fold faster than with the traditional MCTS method.
Improving ML Retrosynthesis Using Deep Learning Tools
In the early days of ML-aided retrosynthesis, algorithms like computer-assisted synthesis planning (CASP) were developed to take a molecular structure as an input and give a list of detailed reaction schemes as an output. Each reaction scheme also listed purchasable starting materials that could create the target molecule through (supposedly) chemically feasible reaction steps.
However, these algorithms failed to gain wide popularity among organic chemists, since they suffered from infeasible suggestions and bias (since human input was necessary).
Subsequently, recent breakthroughs in ML techniques like deep neural networks have significantly improved data-driven synthetic route designs without human intervention. These ‘deep’ networks are artificial decision-makers with multiple hidden layers between the input and output layers, creating a more complex decision tree.
In recent years, a group of scientists developed a CASP application integrated with various portions of retrosynthesis knowledge called“ReTReK” (that apparently is an abbreviation for “retrosynthesis planning application using retrosynthesis knowledge”).
ReTReK is based on a data-driven framework of retrosynthetic predictions by incorporating deep learning with the traditional path search by Monte Carlo Tree Search (MCTS, highlighted above).
What is Deep Learning?
Deep Learning (DL) is an ML technique that teaches computers to do what comes naturally to humans, and is essentially a neural network with three or more “layers”. Each layer behaves as a neuron in our brains, processing an input to produce an output.
While traditional ML algorithms are linear (an input leads to a specific outcome), DL algorithms can build increasingly complex and abstract hierarchies due to multiple processing pathways.
This can come in the form of different forms or architectures, including deep neural networks, deep belief networks, deep reinforcement learning, recurrent neural networks, convolutional neural networks and transformers.
To make a long story short, ReTReK successfully incorporated deep learning as a “knowledge concept” parameter. This meant it could suggest synthetic pathways that looked odd or did not align with traditional organic chemistry.
However, these search results turned out to be more favorable synthetic chemical routes than the ones generated without the knowledge concept (strict rule-based approach). This is akin to game algorithms suggesting a “strange” and “obscure” move that ultimately proved to be game-winning.
The Future of AI-Driven Retrosynthesis
Improving Existing Reaction Databases
We know that reaction databases are critical in AI-driven knowledge-based retrosynthesis, since many models are “trained” using such data sets. Databases that store biosynthesis routes exist, such as:
- MetaCyc5, a curated database of experimentally elucidated metabolic pathways from all domains of life
- KEGG6, a collection of manually drawn pathway maps representing our knowledge of the molecular interaction, reaction and relation networks
Unfortunately, when it comes to complex molecules, these knowledge-based retrosynthesis approaches are often poorly applicable since the reactions of their natural biosynthetic pathways might not be initially known, and hence not included in those databases.
On the other hand, the rule-based models of retrosynthesis approaches (e.g. RetroPathRL, an MCTS reinforcement learning method guided by chemical similarity) can match the target compound to a collection of reaction rules and make predictions.
These rules are either summarized manually by scientists or extracted automatically from the reaction databases. But, even if these rule-based methods have led to promising results, they also have some limitations since
- the formulation of the rules is complicated and time-consuming
- the degree of specificity of these rules can lead to invalid or incomplete proposals, and
- they can’t predict reactions beyond the rule databases.
Fully Data-Driven Retrosynthesis
In contrast to these classical rule-based models, we now have the entirely data-driven toolkit BioNavi-NP, just developed, that can predict the biosynthetic pathways for both natural products (NPs) and NP-like compounds.
Initially, a single-step retrosynthesis prediction model is trained using both general organic and biosynthetic reactions. This is done using a novel architecture (set of rules) that aims to solve sequence-to-sequence tasks while handling long-range dependencies with ease. This is known as an end-to-end transformer neural network, a state-of-the-art technique in the field of natural language processing.
In particular, the BioNavi-NP model uses additional tools, including:
- Data Augmentation, a technique used to increase the amount of data by adding slightly modified copies of already existing data or newly created synthetic data from existing data, and
- Ensemble Learning, combining multiple learning algorithms to obtain better predictive performance. This alone was enough for BioNavi-NP to achieve a top-10 prediction accuracy of 60.6% on a single-step retrosynthesis test, which is 1.7 times more accurate than the previous rule-based models.
AI-Assisted Synthesis Planning in 2023
Finally, just this month (February 2023) a group of researchers made a discovery that can dramatically speed up the planning of future chemical synthesis, providing a proof-of-concept by synthesizing a complex alkaloid found in nature.
The database in question is the SYNTHIA Retrosynthesis Software (owned by Merck), which contains valuable information on chemical synthesis pathways, formulas and even step-by-step methods to create millions of molecules.
By combining the SYNTHIA Retrosynthesis Software with an algorithm they developed to curate all the data, these researchers identified the critical steps in the alkaloid (a large family of naturally occurring molecules) synthetic pathway.
They reached a solution to create the molecule in just 3 steps, much lower than predicted. The researchers confirmed that these routes were of high impact, while also identifying the other feasible but inefficient steps for completing the synthesis.
(If you’d like to learn more about AI/ML retrosynthesis tools, a more detailed list can be found here and here)
AI in Automation of Chemical Synthesis
We’ll end off this topic by walking you through automated synthesis, a set of techniques that use robotic equipment to perform chemical synthesis, which can also be AI-assisted.
Fully automated chemical synthesis using AI robots, instead of humans, is a future megatrend of Industry 4.0 (or the fourth industrial revolution). Industry 4.0 refers to the intelligent, connected production systems that are designed to sense, predict and interact with the environment, to make decisions that support production in real-time.
For example, IBM’s RoboRXN is a cloud-based AI-driven lab that efficiently automates most of the initial groundwork in materials synthesis. Behind the RXN is a state-of-the-art neural ML translation method that can predict the most likely outcome of a chemical reaction using neural machine translation architectures.
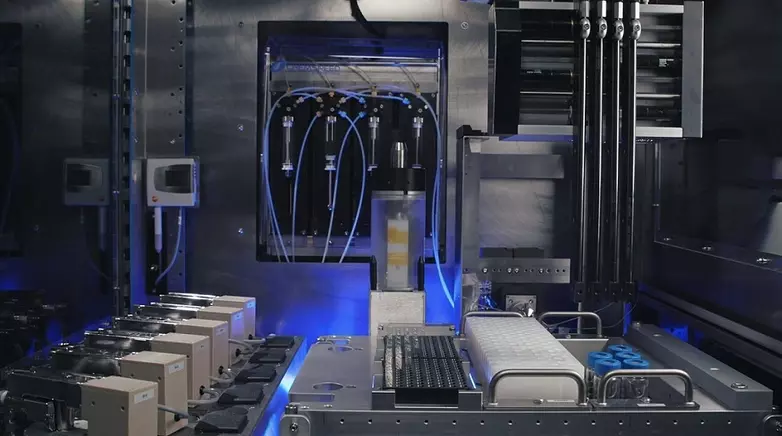
In particular, the architecture used translates the language of chemistry converting reactants and reagents to products utilizing the SMILE representation, which translates a chemical’s three-dimensional structure into a string of symbols that is easily understood by computer software.
Interestingly, the RXN robot has also integrated a retrosynthetic architecture using as a prediction model the Molecular Transformer, which is an ML model inspired by language translation that accurately predicts the outcomes of organic reactions and estimates the confidence of its own predictions. Not bad!
Industry 4.0 represent an industrial shift that blurs the lines between the physical, digital and biological worlds. Still in its early stages, much is needed to improve AI-driven automated synthesis, including reducing cost, implementing standardization and boosting efficiency and scale.
AI Startups Involved in Chemical Synthesis
Here are some startups and are developing AI/ML tools to improve chemical synthesis and retrosynthesis processes:
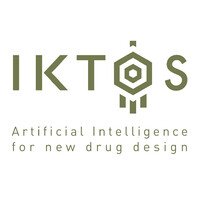
Iktos (@IktosAI) offers AI solutions applied to chemical research such as Spaya—a synthesis planning software based upon Iktos’s proprietary AI technology for retrosynthesis—and Spaya API—a high throughput synthetic accessibility scoring tool for virtual molecule libraries.
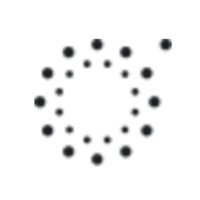
Molecule.one (@MoleculeOne)utilizes AI to predict chemical reactions with unprecedented accuracy. They offer RetroM1—an AI-powered retrosynthesis pathway planner—and M1RetroSAS—a tool to screen tens of thousands of compounds for synthetic accessibility.
Chemical.ai (@chemical_ai) offers the ChemFamily products, including ChemAIRS, ChemAIOS, ChemAIoT and ChemAILab, based on its proprietary retrosynthesis algorithm as a standard closed data loop to enhance chemical synthesis efficiency.
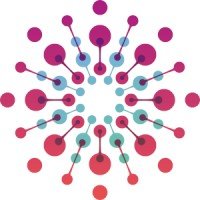
Chemify aims to digitize chemistry and produce solutions to run chemical code for chemical and drug discovery, chemical synthesis and materials discovery.
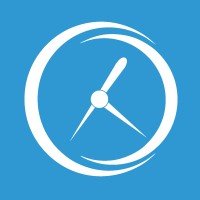
Pending.ai (@PendingAI) utilizes AI to learn chemistry from a database of more than 130 million compounds, 20 million reactions and 146,000 proteins, allowing researchers to generate novel molecules with neural networks, do structure-based drug design, plan chemical synthesis and conduct high-throughput chemistry. Elsevier is collaborating with Pending.AI to develop a predictive retrosynthesis tool based on deep learning.
Reference
Zhong, Z., Song, J., Feng, Z., Liu, T., Jia, L., Yao, S., … & Song, M. (2023). Recent advances in artificial intelligence for retrosynthesis. arXiv preprint arXiv:2301.05864.
About the Author
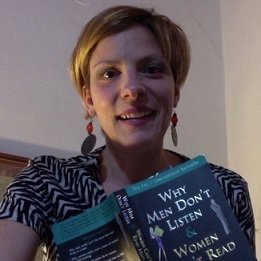
Marina is a molecular and cellular biologist, writer and a bit of an intellectual still trying to fit in. She has 20+ years of experience in academia, startups and MNCs. Currently is a life science consultant. She writes about A.I., drug discovery and biotech.